(Workshop) AI4Mat NeurIPS 2022 Workshop
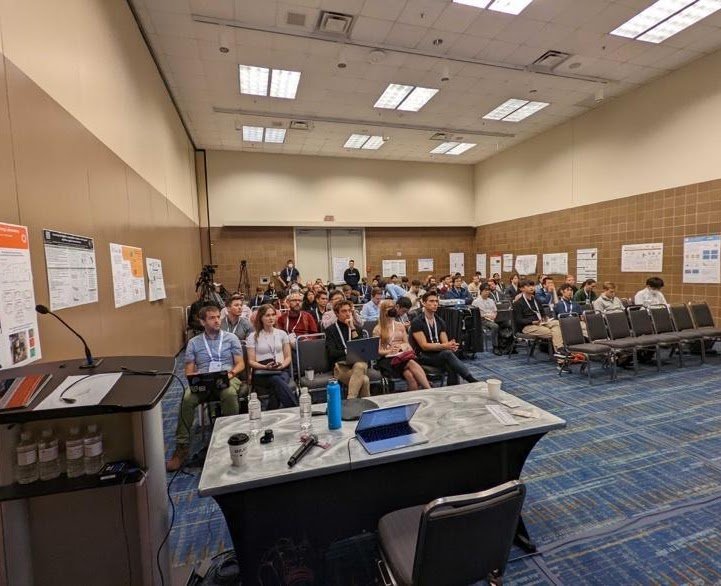
I had the chance to take part in the AI4Mat NeurIPS 2022 Workshop at NeurIPS 2022. It was a cross over of ML researchers and people in material science, the ideal breeding ground for new ideas of how to perform better material science.
Specific work that stood out to me was for example:
More trustworthy Bayesian optimization of materials properties by adding humans into the loop
This paper poses a simple challenge: How do we integrate experts into the material optimisation loop? More specifically, can we effectively use them to judge sample quality? This is a challenge we are familiar with through our recommendations we make to material experts. Oftentimes, due to the nature of the search strategy of bayesian optimisation, we face candidate experiments that experts can exclude from first principle, as e.g. that certain combination of factors never synthesis.
Adding a humans into the loop can be useful if their feedback is reliable and can be integrated effectively. This workshop paper inserts the human in the sample quality check, asking the expert to "to comment on the sample quality, which results in more trustworthy BO results." Convergence of BO is a key challenge in materials optimisation and adding one more layer of assurance at relative low cost is a reasonable idea.
I think this paper starts an important discussion and highlights the continuing importance of expert knowledge in the automated optimisation process. While more data is in principle a good thing for any learning algorithm, the fact only holds that "garbage in, garbage out". Integrating expert knowledge is a crucial step to a successful, and usually faster, search strategy.
A Self-driving Laboratory Optimizes A Scalable Process For Making Functional Coatings
I am discussing this paper in more detail in this blog entry, but find it worth mentioning here once more again. We have seen quite a few examples of 'self-driving laboratories' over the last years, all at different scales, solving different optimisation tasks. I enjoyed this paper as it is honest about the true complexity of automated experimentation. It also shows evidence for scale-up of the optimal material combination they found: "The champion coating conditions were scaled up to an 8× larger area using the same spray-coating apparatus with no further optimization and no reduction in coating quality or conductivity." This is not always the case, and certainly depends on the material at hand. For example, in biosynthetic materials, key challenges include the scale-up of production and making sure that the properties achieved at small scale, also exist at bigger production scales.
Read on more in this blog entry.
I am very happy with this workshop which enabled very useful conversations on the interface of Machine Learning and Material Science. I do hope that there will be many more workshops like this as the challenges discussed barely scratch the surface on the problem space of Machine Learning applied to Material Science.
Jakob Zeitler, Research Scientist at Matterhorn Studio
Get in touch
We will happily guide you through the emerging space of data-driven material discovery. We look forward to learn from your experience and problem space.